Lead Scoring Through ML-Based Propensity Modeling
Posted by | Fuld & Company
An enterprise cloud solution provider wanted to increase sales conversions by better prioritizing leads.
Objective
To configure, test, and deploy an ML-based propensity model that would prioritize generated leads based on propensity and help drive conversions for the sales team.
Approach
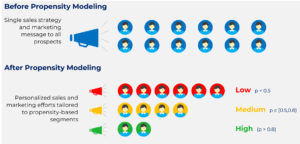
Propensity modeling enables the sales team to personalize and target efforts
- Understood the current scoring scheme and its limitations.
- Collected data for all leads from CRM, web portal, 3P lead partners, and 3P company databases.
- Trained and tested ML classification models on historical customer win/ loss data.
- Tested and used the best-performing algorithm among logistic classification, Random Forest, ensemble classification, and neural networks.
- Clustered leads and created targetable sales plays for the sales and marketing teams
Algorithms used include: classification models such as logistic classification, Random Forest, ensemble classification, and neural networks.
Outcome
- Visualization of classification outcomes and analyses on a Tableau dashboard
- Integrated classification model with client CRM to identify leads with high probability of conversion
- Increased conversion rate by 11%